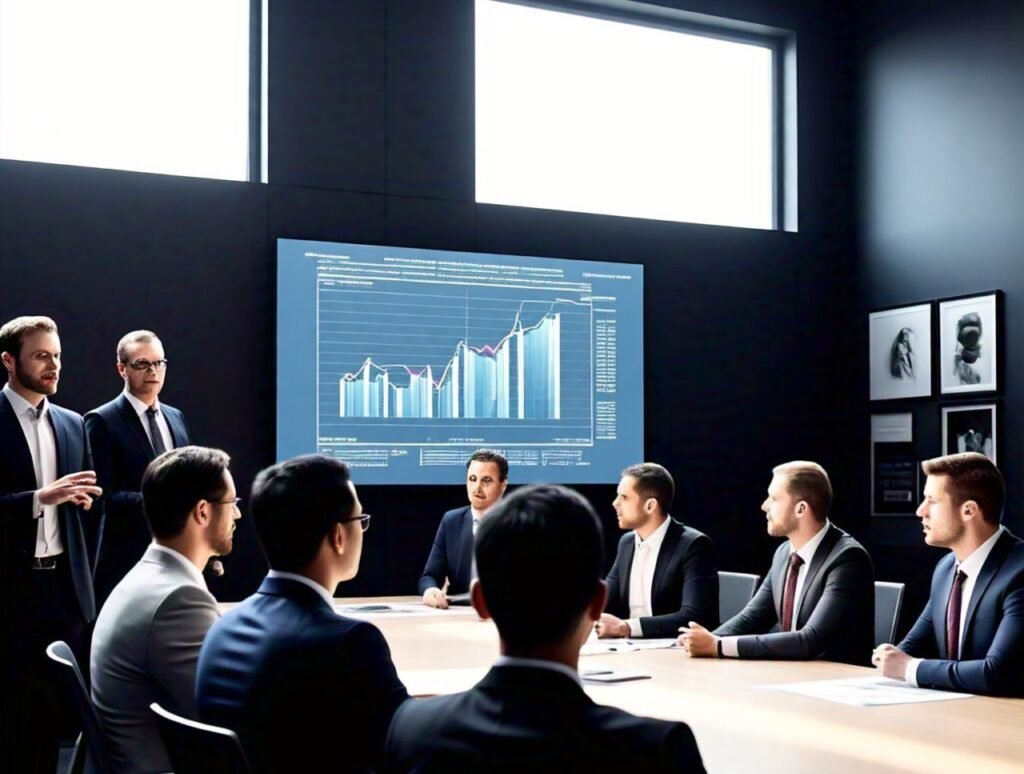
Key Takeaways:
- AI enhances traditional BI by enabling faster data analysis, predictive insights, and automation.
- Key technologies like machine learning, NLP, and neural networks play a crucial role in improving BI systems.
- Integrating AI into BI requires proper data management, assessment of current tools, and addressing challenges such as skills gaps and data privacy concerns.
Introduction to AI in Business Intelligence
Artificial Intelligence (AI) is transforming the landscape of Business Intelligence (BI). By integrating AI, businesses can efficiently process vast amounts of data, turning raw information into valuable insights. Traditional BI systems often struggle with large datasets, but AI uses advanced algorithms to identify patterns and trends that human analysts may miss, thus enabling faster, data-driven decisions.
AI in BI includes machine learning, predictive analytics, and natural language processing (NLP). These technologies allow businesses to uncover complex data trends, enhance decision-making, and maintain a competitive edge. This guide will explore how AI is integrated into BI systems and the benefits it offers.
Understanding Business Intelligence
Business Intelligence (BI) is a set of technologies that help businesses gather, analyze, and present data for decision-making. Key BI components include data warehousing, data mining, reporting tools, and predictive analytics. While traditional BI methods excel in organizing data and visualizing trends, they often fall short in real-time analysis and pattern recognition. AI addresses these limitations, providing businesses with the agility and accuracy needed to stay ahead in a data-driven world.
Benefits of Integrating AI in Business Intelligence
- Enhanced Data Analysis: AI can process and analyze large datasets at speed, identifying hidden patterns and offering insights that traditional methods may overlook.
- Predictive Analytics: Machine learning models can forecast future trends based on historical data, helping businesses anticipate market changes and optimize resources.
- Automation: AI automates repetitive tasks like report generation, saving time and reducing errors while improving efficiency.
- Improved Customer Insights: By analyzing unstructured data from sources like social media, AI offers deeper insights into customer behavior, enhancing product offerings and customer experiences.
Key AI Technologies for Business Intelligence

- Machine Learning: AI uses algorithms to analyze data, uncover trends, and make predictions. For example, retail businesses can forecast sales trends and manage inventory better using machine learning.
- Natural Language Processing (NLP): NLP enables machines to understand and interact with human language. It can be used in BI tools for analyzing customer feedback and automating interactions.
- Neural Networks: These networks mimic the human brain to recognize patterns in complex data, such as images or text. Businesses use neural networks to improve customer service or analyze social media for sentiment analysis.
Steps to Integrate AI into Existing BI Systems
- Assessment: Review current BI capabilities and data sources to identify gaps where AI can add value.
- Define Objectives: Clearly outline the goals for AI integration, such as enhancing analysis speed or automating tasks.
- Choose the Right Tools: Select AI tools that are compatible with existing BI systems and meet specific needs like machine learning or NLP.
- Data Quality and Preparation: Clean and structure data to ensure that AI tools can provide meaningful insights.
Data Management and Preparation for AI Integration
Effective AI integration depends heavily on high-quality data. Organizations must clean, organize, and structure data from various sources, ensuring that it is accurate and consistent. Well-prepared data boosts AI’s effectiveness, enabling better predictions and insights in BI systems.
Challenges in AI Integration for Business Intelligence
- Skills Gap: A shortage of skilled employees may hinder AI adoption, necessitating training and development programs.
- Data Privacy: With large volumes of data, businesses must ensure that they comply with data privacy regulations.
- Technological Limitations: Outdated systems may not support AI tools, requiring infrastructure upgrades for effective integration.
- Resistance to Change: Employees may resist AI integration due to fear of job loss, requiring effective change management strategies.
Future Trends in AI and Business Intelligence
The future of AI in BI will see advancements in real-time analytics and machine learning algorithms, providing businesses with faster, more accurate insights. AI tools will become more accessible, empowering non-technical users to leverage data in decision-making, thus democratizing access to advanced analytics.
Ethical Considerations in AI-Driven Business Intelligence
While AI offers immense potential in business intelligence, its implementation also brings up several ethical considerations that organizations must address. The use of AI in BI systems requires careful thought about data privacy, transparency, and fairness to avoid unintended consequences that could harm both the business and its customers.
Data Privacy and Security
One of the most pressing ethical concerns in AI-driven BI is the handling of sensitive customer and business data. AI systems rely on large datasets to identify patterns, predict trends, and generate insights, but the collection and processing of this data must adhere to strict privacy regulations. Organizations need to ensure that they are transparent about data collection methods, informing customers about what data is being collected and how it will be used. Failure to adhere to data protection laws, such as GDPR, can result in significant penalties and loss of consumer trust.
Bias in AI Algorithms
AI systems are only as good as the data fed into them. If the data contains biases, the resulting algorithms can perpetuate those biases, leading to skewed analyses and unfair outcomes. For example, if a recruitment AI system is trained on biased hiring data, it might unintentionally favor certain demographic groups over others. To mitigate this, organizations must ensure that their AI algorithms are trained on diverse and representative datasets, regularly audited for bias, and continuously improved over time. A key part of implementing ethical AI is fostering diversity both in data and in the teams creating these AI models.
Transparency and Accountability
With AI becoming more integral to decision-making, organizations must ensure transparency in how their AI systems work and the decisions they make. AI models, particularly machine learning models, can sometimes function as “black boxes,” meaning their decision-making processes are not always easily interpretable. Businesses need to prioritize explain ability in their AI systems, ensuring that the reasoning behind important decisions can be understood and justified. This is especially critical when AI is used for high-stakes decisions, such as determining credit scores, hiring decisions, or customer service outcomes.
The Role of Human Oversight
While AI can automate many aspects of business intelligence, human oversight remains crucial. AI models should be viewed as tools that enhance human decision-making, not replace it. For instance, an AI system may identify a potential market trend, but it’s up to human decision-makers to interpret these insights in the context of broader business goals. By maintaining a balance between AI automation and human input, organizations can ensure that they are using AI in a way that aligns with their ethical standards and broader corporate values.
Also read: Creating a Winning Business Case for Facility Investments
AI in Business Intelligence for Small and Medium Enterprises (SMEs)

While large enterprises have traditionally been the primary adopters of AI in business intelligence, small and medium-sized enterprises (SMEs) are also beginning to recognize the value that AI can bring to their operations. AI-powered BI tools are increasingly becoming more accessible, allowing smaller organizations to compete with larger players in terms of data analysis and decision-making.
Affordable AI Solutions for SMEs
The cost of AI technology has historically been a barrier for many SMEs, but this is changing rapidly. Many vendors now offer scalable, cloud-based AI solutions that allow smaller businesses to access powerful analytics tools without the need for significant upfront investment. These platforms often come with user-friendly interfaces, making it easier for non-technical staff to use and gain insights from AI-driven BI tools.
Improved Customer Targeting
AI-driven BI systems can significantly enhance the ability of SMEs to understand their customers. By analyzing customer data, businesses can identify purchasing patterns, predict future behaviors, and tailor marketing strategies to individual needs. This level of personalization can help SMEs compete with larger companies that typically have more resources at their disposal for market research and customer engagement.
Operational Efficiency and Cost Savings
For SMEs, operational efficiency is key to remaining competitive. AI can help streamline processes, optimize inventory management, and even automate tasks that were once time-consuming and prone to human error. By improving efficiency, SMEs can reduce costs, enhance their profitability, and focus on strategic initiatives to grow their business.
Decision-Making Support
One of the biggest challenges SMEs face is making informed decisions with limited resources. AI-driven BI tools can help by providing real-time insights and predictive analytics, giving business owners a clearer picture of their operations. With data-backed insights, SMEs can make more confident decisions about pricing, staffing, marketing, and other business aspects, enabling them to respond quickly to market changes.
Conclusion and Final Thoughts
The integration of AI in business intelligence is a transformative strategy for organizations seeking to enhance their data analysis and decision-making processes. By leveraging AI, businesses can automate tasks, predict future trends, and gain deeper insights into customer behavior, enabling them to remain competitive in a data-driven world.
Investing in AI for BI isn’t just about upgrading technology—it’s about reshaping how organizations approach data and decision-making. Companies that embrace AI-driven BI systems will gain significant advantages in agility, operational efficiency, and market positioning.
FAQs:
What is the role of AI in Business Intelligence?
AI improves data analysis, automates tasks, and provides predictive insights, helping businesses make smarter, data-driven decisions.
How does machine learning benefit Business Intelligence?
Machine learning analyzes large datasets to uncover hidden patterns, forecast trends, and support better decision-making.
What are the challenges in integrating AI with BI systems?
Challenges include a skills gap, data privacy concerns, technological limitations, and resistance to change within the organization.
How can businesses prepare for AI integration?
Businesses should assess their current systems, define AI goals, choose the right tools, and ensure data quality and preparation.